Edge Intelligence: Utilizing Al Technology at the Network
Edge Intelligence (EI) is an emerging technology that refers to the utilization of the Artificial Intelligence paradigm in Edge computing scenarios. It enables machines to make decisions on locally harvested data. It doesn’t send it to a centralized cloud or on-premise server. This offers advantages such as low latency, enhanced privacy, scalability, diversity, and increased reliability. The demand for EI is fueled by the proliferation of sensors and smart devices generating multi-modal data (audio, pictures, video). Various sectors, like healthcare, augmented reality, industrial automation, network security, autonomous vehicles, smart home automation, etc., can enhance their performance using edge intelligence.
Introduction to Edge Intelligence
It is an emerging technology that refers to the utilization of the Artificial Intelligence (AI) paradigm in Edge Processing Scenarios.
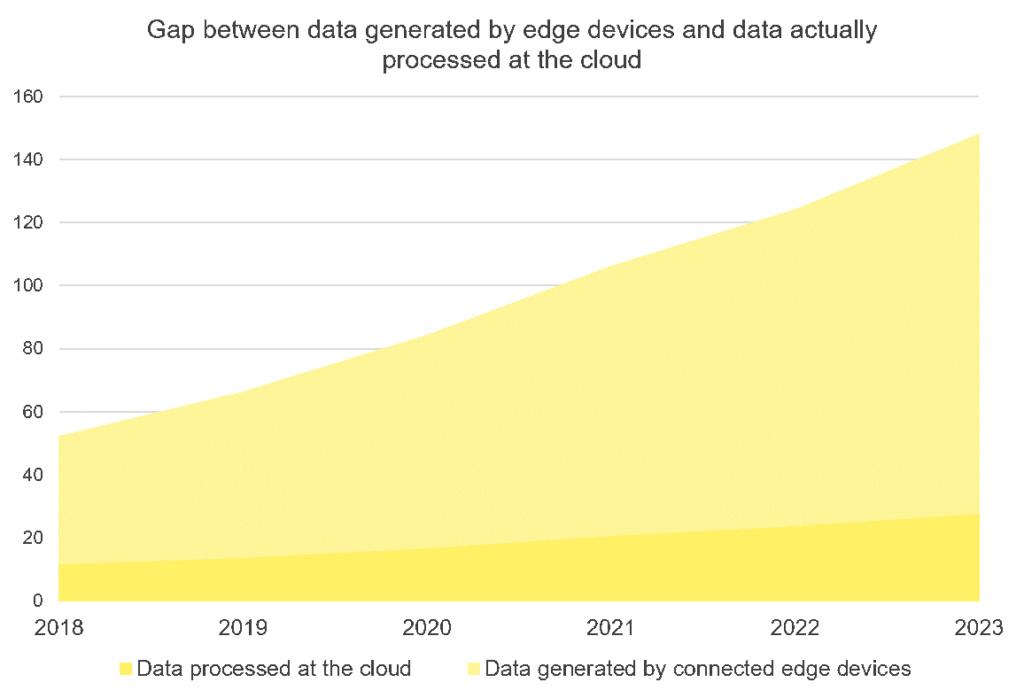
Edge intelligence technology refers to a set of connected systems and devices for data collection, caching, processing, and analysis in proximity to where data is captured based on artificial intelligence. It aims to enhance data processing and protect the privacy and security of data and users. The increasing number of IoT and mobile devices lead to the generation of vast volumes of data. Deploying intelligent algorithms at the edge can help quickly analyze huge volumes of data and extract insights for decision-making purposes. Figure 1 shows the gap between the amount of data generated and the amount of data processed, indicating the need to adopt edge intelligence.
Benefits of Edge Intelligence
Using AI algorithms at the edge of the network offers various advantages:
- Latency – The training algorithms are deployed close to the user’s device. This significantly reduces the cost and latency of processing. Thus, it is beneficial in providing faster processing and computation of the data received from the devices.
- Privacy – The data required for learning applications is stored locally on the edge device because it does not store the data at any third-party location, the privacy and security of the user’s device remains unreachable.
- Scalability—Owing to richer data and application scenarios, EI has the potential to drive widespread adoption of deep learning and artificial intelligence across multiple industries.
- Cost—The edge AI applications can reduce the requirement for energy consumption and infrastructure development through their utility, thus saving users money.
- Reliability—With the introduction of EI, the reliability level of computational operations will improve. It’ll follow the decentralized and hierarchical computing architecture, enabling the user’s device to calculate the data. With deep learning and artificial intelligence getting involved in the processing, the computation will be more reliable and secure compared to the existing computation models.
Levels of Edge Intelligence
Although EI offers many advantages, there are many constraints in realizing its full potential, such as:
- Resource Constraint – AI algorithms have high computational and memory requirements, which are lacking in edge devices that have limited resources.
- Data Scarcity at the Edge – Data collected at the edge is sparse and unlabelled, which is a challenge for implementing machine learning algorithms as they require high-quality training instances.
- Data Inconsistency – Data is collected from several resources for different applications, which leads to different sensing environments and sensor heterogeneity problems.
- Bad Adaptability of Statically Trained Model – The statically trained models at the cloud cannot effectively deal with the unknown new data and tasks in unfamiliar environments at the edge, which results in low performance.
Owing to the above challenges, EI functions in a cloud-edge-device coordination manner via data offloading. Based on the amount and path length of data offloading, EI is rated into six levels, as shown in Figure 2.
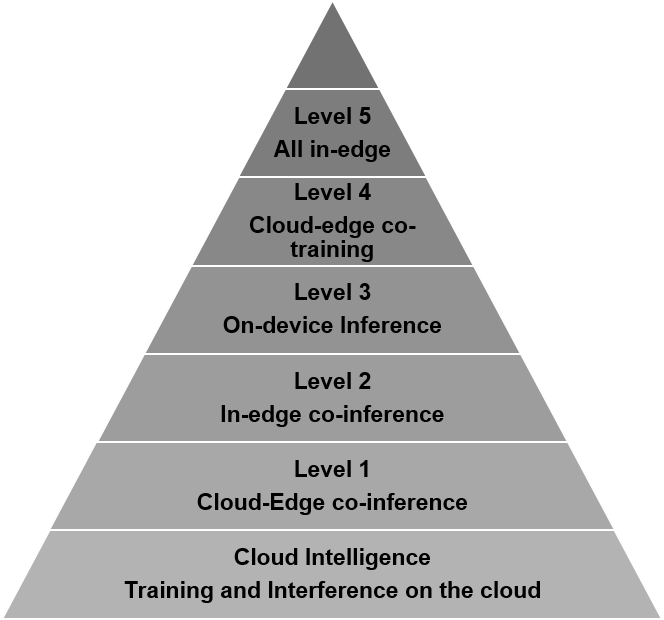
Various Levels Defined
Cloud Intelligence – Training and Inference of AI models fully in the cloud
- Level 1: Cloud–Edge Co-inference and Cloud Training – The model is trained in the cloud, however, it is implied in an edge-cloud cooperation manner.
- Level 2: In-Edge Co-inference and Cloud Training – The model is trained in the cloud but inferred at the edge.
- Level 3: On-Device Inference and Cloud Training – The model is trained in the cloud but is inferred in a fully local on-device manner.
- Level 4: Cloud–Edge Co-training and Inference – The model is trained and inferred in the edge-cloud cooperation manner.
- Level 5: All In-Edge – The model is trained and inferred at the edge.
- Level 6: All on-device – The model is trained and inferred on the devices.
This conflict indicates that there is no “best level” for the deployment of EI. Instead, the “best level” depends on the application at hand and is determined by jointly considering multiple parameters, including latency, energy efficiency, privacy, and WAN bandwidth cost. As the level of EI increases, the amount and path length of data offloading reduces, thus reducing transmission latency and bandwidth costs and increasing data privacy. However, this is achieved at the cost of increased computational latency and energy consumption.
Applications of Edge Intelligence
Edge intelligence can enhance the performance of various sectors, such as healthcare, augmented reality, industrial automation, network security, autonomous vehicles, and smart homes.
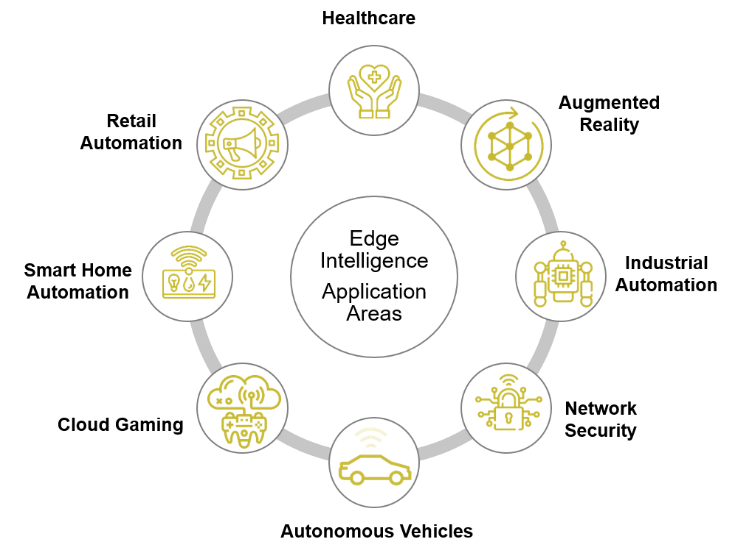
- Healthcare – Edge Intelligence will support real-time analysis and inferencing of crucial data.
- Augmented Reality – The introduction of edge intelligence will enhance computation speed. It will remove the patches in interaction with the virtual environment and improve the user experience.
- Industrial Automation – Edge Intelligence will help provide faster responses to sensor data, which will help increase production.
- Retail Automation – Faster processing using edge intelligence will decrease latency in payment options or transactions. It will prove to be a revolution in retail automation.
- Smart Homes – With smart home automation, major issues arise because of the delay in decision-making. Moreover, introducing edge intelligence in smart homes will decrease data processing time and provide faster decision-making.
- Autonomous Vehicles – The real-time processing of environmental conditions data will help reduce the chances of accidents and increase precision in autonomous vehicles, which will be more secure.
- Live Gaming – Edge Intelligence will reduce latency in live gaming and take the experience to a whole new level.
- Network Security – The introduction of edge intelligence in the network will provide security to edge devices and data stored in cloud networks. There will be faster and better security solutions in case of any threat or attack.
Startups and Companies Leading the Way in Edge AI
- Databricks: Established in 2013, our company offers a comprehensive analytics platform that combines various data sources into a unified system.
- The Not Company: Founded in the year 2020, an artificial intelligence model was developed to create plant-based alternatives to meat-based recipes.
- Gridware: A startup’s primary objective is to develop hardware and software solutions that effectively mitigate and identify potential causes of wildfires.
- Axelera AI: In 2019, Metis AI platform was established to offer AI solutions at the edge.
- Cybereason: Established in 2015, we present a fresh perspective on cybersecurity, providing a novel approach to safeguarding digital assets.
- Accern: A no-code development platform is revolutionizing AI workflows for enterprises, catering to renowned organizations like Allianz, IBM, and Jefferies and expediting their operations.
- Shield AI: Utilizing research and expertise to create state-of-the-art artificial intelligence software and systems.
Conclusion
Edge Intelligence will propel technology companies toward the next generation of connectivity and operational efficiency. The intelligent edge will support large-scale transformational solutions across major industry verticals. For instance, it can change the supply chain into a programmable, responsive, and adaptable digital network. Moreover, it can restructure itself in response to shifting demands and disturbances. Further, AI-enabled drones can be deployed to monitor the risk posed by aging infrastructure or inspect pipelines for defects.
Moreover, addressing several obstacles is essential before the full potential of edge intelligence can be realized. Academic institutions and businesses are also looking for solutions to these problems. Also, the resource constraint can be circumvented by quantizing or pruning neural network models, reducing the memory footprint and hardware power consumption in neural networks. Furthermore, digital twins can be used to realize network edge inference capabilities.