Transformative Potential of Generative AI in MedTech
A new branch of artificial intelligence, called Generative AI or Gen-AI, has recently evolved and is rapidly being adapted across industries. Gen-AI represents a significant update over conventional AI. Unlike conventional AI, which utilizes predictive models to make predictions, Gen-AI can create content and synthetic data in real time. This capability has propelled Gen-AI to be leveraged by multiple industries across their workflows. The article will delve deeper into generative AI in MedTech and its revolutionary capabilities impacting the domain. Also, it will highlight how Gen-AI is being incorporated from the product’s research and development phase to corporate functions, the advantages of Gen-AI, its industrial use cases, and the challenges associated with it.
What is Gen-AI?
Generative AI operates based on sophisticated machine learning techniques, particularly within generative models. The process comprises learning intricate patterns from preexisting data and utilizing this acquired knowledge to create novel and distinct outputs. Gen-AI’s innovative aspects lie in its proficiency in producing content of a highly realistic and intricate nature, mirroring human creativity.
Recently, multiple trained models have come out that can generate various content. For example, Open-AI’s GPT-3 (text generation), GitHub’s Co-pilot X (code generation), and NVIDIA’s StyleGAN (image generation).
How Can MedTech Leverage Gen-AI?
One of the most prominent challenges in incorporating Gen-AI is knowing how and the area of application to implement it. Due to its diversified applications, it’s important to identify and prioritize the right areas for its implementation. Here are a few areas;
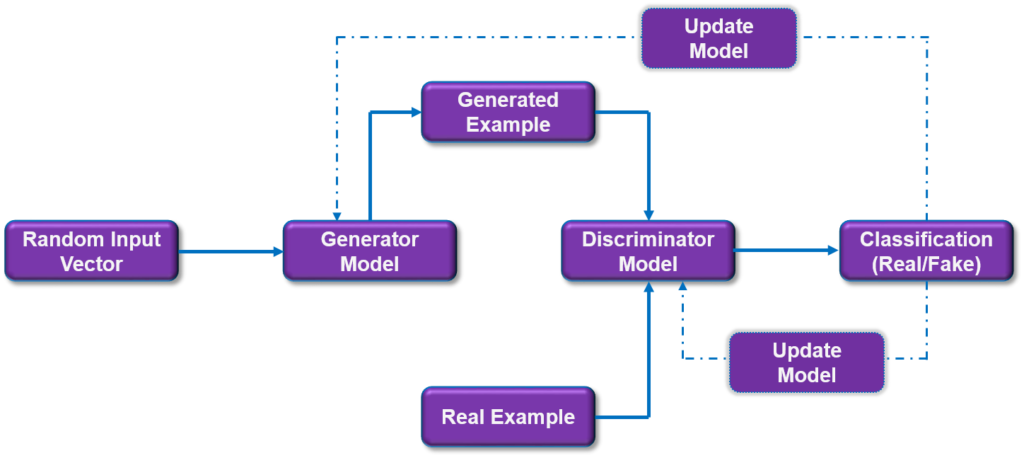
Figure 1. Generative AI in the MedTech output process
- Research and development: MedTech software-enabled products have higher product development costs. Gen-AI can support software development by generating codes, documenting codes, and generating test cases. Additionally, it can help design a new product and can support documentation & review work for clinical trial reporting.
- Operations: Streamlining operations across the value chain is critical for ensuring optimum output. The ability of Gen-AI to generate synthetic data, which could be utilized to train machine learning models, can be incorporated to improve quality in the production process. The systems trained with this synthetic data can identify product flaws. Additionally, Gen-AI could be used for procurement as well.
- Marketing & Sales: Gen-AI solutions can help salespeople automatically document their visits, capture and analyze customer information, and generate insights that support the next interaction.
- After-sales & customer support: Gen-AI can be leveraged to reduce manual and time-consuming tasks. Interacting with customers is advantageous, as it can offer better customer service since it already has the necessary information about the customer.
- Corporate functions: Gen-AI can boost collaboration and productivity amongst teams. It can streamline multiple reports and generate more actionable insights and outputs.
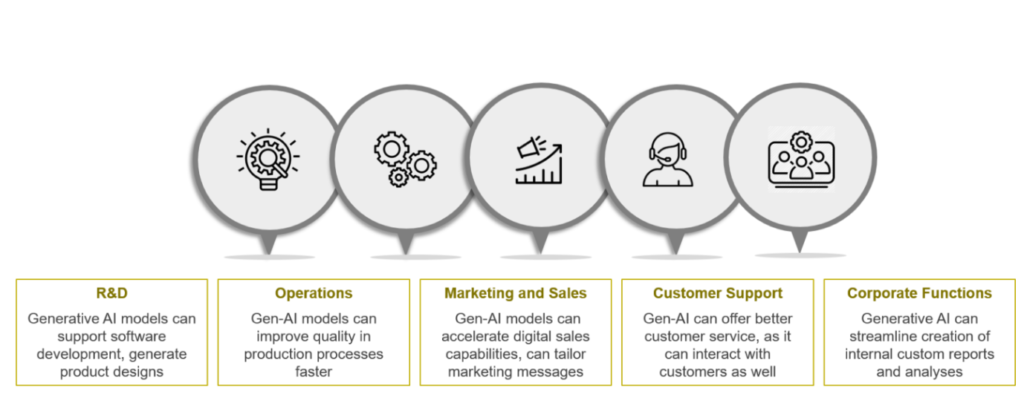
Figure 2. Incorporating Gen-AI across MedTech
Generative AI in MedTech Use Cases
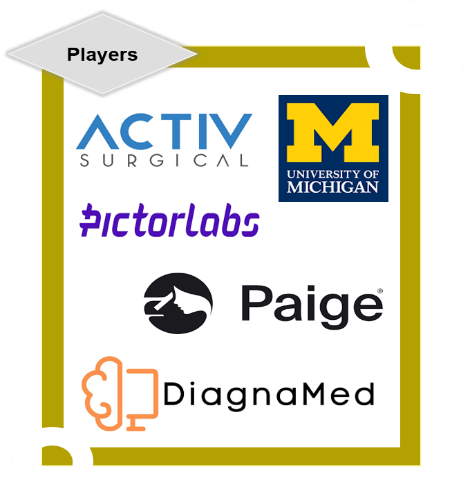
Figure 3. Few Players Leveraging Gen-AI in MedTech Industry
Gen-AI can potentially shape how medical devices or services are provided. Below are some of the industrial use cases wherein Gen-AI has been incorporated within medical services.
- DiagnaMed has recently launched a Gen-AI medical chatbot, Dr. GenAI. The chatbot can obtain personalized medical conditions from a patient very quickly. Dr. GenAI can learn about a person using their medical information, such as physical traits, vitals, and lab test results.
- Paige and Pictor labs have incorporated Gen-AI to enhance image quality. In addition, the Paige Platform enhances doctors’ capabilities beyond the limits of traditional pathology. It promotes workflow efficiency and harnesses the precision of Gen-AI in cancer diagnosis.
- The University of Michigan has developed a Gen-AI model capable of simulating case scenarios during sepsis treatment
- Active Surgical has incorporated Gen-AI for intraoperative guidance during surgical workflows. The advanced visualization platform assists surgeons in better-informed decision-making.
Generative AI in MedTech: Challenges
Incorporating Generative Artificial Intelligence (Gen-AI) into the med-tech industry presents several challenges highlighted below:
- Regulatory compliance: The MedTech industry is highly regulated. Incorporating Gen-AI, may require adherence to regulatory frameworks that have not come up yet, although FDA does have guidelines for AI/ML-enabled devices. As of now, there are no FDA-approved medical devices enabled by Gen-AI.
- Ethical Consideration: Artificial intelligence suffers from biases. As a result, Gen-AI applications may raise ethical concerns, especially when dealing with critical healthcare decisions.
- AQ2Costs: Gen-AI is projected to grow faster in health care than any other industry; it could reach a total market size of $21 billion by 2027. However, implementing Gen-AI in MedTech may involve high upfront costs for technology acquisition, training, and ongoing maintenance.
- Generation of Fake data sets: LLMs or Language learning models have recently been found to generate fake datasets to support scientific hypotheses. This capability can be employed by a “researcher or a team of researchers to fabricate measurements for imaginary patients or generate an extensive dataset for medical devices testing involving animals.”
Cybersecurity: Gen-AI applications in med-tech will raise new security and cybersecurity concerns, which are already well-established for healthcare companies. Additionally, unauthorized access to AI systems can potentially lead to the manipulation of medical data, which can compromise patient privacy and influence treatment decisions.
Conclusion
The integration of Gen-AI can potentially significantly influence the landscape of medical devices and services. For med-tech, the main advantages of leveraging Gen-AI are increased efficiency by reducing manual processes, enhanced personalization with customers & improvements in existing product designs. Also, several industrial use cases highlight the diverse applications of Gen-AI within the medical sector. Notable examples include Dr. Gen-AI chatbot for personalized medical conditions, Paige and Pictor Labs using Gen-AI to enhance pathology image quality. However, it has its own challenges when it comes to regulatory compliance. Determining accountability and liability in cases of Gen AI-generated errors or false positives poses a significant ethical challenge. Additionally, establishing responsibility for adverse outcomes becomes complex when AI systems are involved, potentially leaving patients without clear recourse. Moreover, Gen-AI has strong potential to help med-tech companies work efficiently, smarter, and faster and create value for companies ambitious enough to apply them.